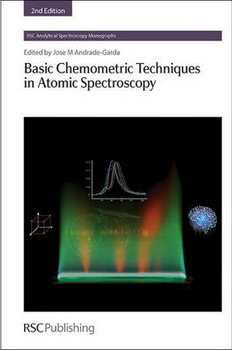
Basic Chemometric Techniques in Atomic Spectroscopy
[BOOK DESCRIPTION]
The first edition of this book was a first book for atomic spectroscopists to present the basic principles of experimental designs, optimization and multivariate regression. Multivariate regression is a valuable statistical method for handling complex problems (such as spectral and chemical interferences) which arise during atomic spectrometry. However, the technique is underused as most spectroscopists do not have time to study the often complex literature on the subject. This practical introduction uses conceptual explanations and worked examples to give readers a clear understanding of the technique. Mathematics is kept to a minimum but, when required, is kept at a basic level. Practical considerations, interpretations and troubleshooting are emphasized and literature surveys are included to guide the reader to further work. The same dataset is used for all chapters dealing with calibration to demonstrate the differences between the different methodologies. Readers will learn how to handle spectral and chemical interferences in atomic spectrometry in a new, more efficient and cost-effective way.
[TABLE OF CONTENTS]
List of Contributors xvii
Chapter 1 An Overview of Atomic 1 (51)
Spectrometric Techniques
Alfredo Sanz-Medel
Rosario Pereiro
Jose Manuel Costa-Fernandez
1.1 Introduction: Basis of Analytical 1 (2)
Atomic Spectrometric Techniques
1.2 Atomic Optical Spectrometry 3 (16)
1.2.1 Classification of Techniques: 4 (5)
Absorption, Emission and Fluorescence
1.2.2 A Comparative View of Basic 9 (7)
Instrumentation
1.2.3 Comparative Analytical 16 (3)
Performance Characteristics and
Interferences
1.3 Atomic Mass Spectrometry 19 (13)
1.3.1 Fundamentals and Basic 21 (3)
Instrumentation of Inductively Coupled
Plasma-Mass Spectrometry
1.3.2 Analytical Performance 24 (4)
Characteristics and Interferences in
ICP-MS
1.3.3 Isotope Ratio Measurements and 28 (4)
Their Applications
1.4 Flow Systems with Atomic Spectrometry 32 (9)
Detection
1.4.1 Flow Injection Analysis and 32 (3)
Atomic Spectrometry
1.4.2 Chromatographic Separations 35 (3)
Coupled On-line to Atomic Spectrometry
1.4.3 Detection of Fast Transient 38 (3)
Signals
1.5 Direct Analysis of Solids by 41 (5)
Spectrometric Techniques
1.5.1 Direct Elemental Analysis by 42 (3)
Optical Spectrometry
1.5.2 Direct Elemental Analysis by Mass 45 (1)
Spectrometry
1.6 Quality Control and Troubleshooting 46 (6)
References 48 (4)
Chapter 2 Classical Linear Regression by 52 (71)
the Least Squares Method
Jose Manuel Andrade-Garda
Alatzne Carlosena-Zubieta
Rosa Maria Soto-Ferreiro
Javier Teran-Baamonde
Michael Thompson
2.1 Introduction 52 (5)
2.1.1 Defining Calibration 54 (3)
2.2 The Least Squares Criterion 57 (4)
2.2.1 Basic Assumptions of the Least 58 (3)
Squares Method
2.3 The Least Squares Fit 61 (5)
2.3.1 Planning Standardization 63 (2)
2.3.2 Setting the Working Range 65 (1)
2.4 Validation of the Least Squares Fit 66 (12)
2.4.1 The Correlation Coefficient 66 (4)
2.4.2 Residuals and Outlying Data 70 (4)
2.4.3 Tests for Equal Variances 74 (1)
2.4.4 Tests for Linearity 75 (3)
2.5 Estimating Unknown Concentrations 78 (6)
2.6 The Standard Additions Method (SAM) 84 (5)
2.6.1 Description of the Method: 84 (2)
Advantages and Disadvantages
2.6.2 Interpolation or Extrapolation 86 (3)
2.7 Practical Application 89 (14)
2.7.1 Experimental Design 89 (5)
2.7.2 Effect on the Predictions and 94 (7)
Confidence Intervals
2.7.3 Comparison of the Predictions and 101 (2)
Confidence Intervals Obtained with
Interpolation and Extrapolation
2.8 Polynomial Regression 103 (4)
2.8.1 Over-fitting 104 (1)
2.8.2 Shape of the Polynomial 105 (2)
2.9 Appendix 1. Mandel's Test to Check 107 (6)
for Linearity
Comparing the Two Definitions 110 (3)
2.10 Appendix 2. Comparison of Two 113 (10)
Regression Lines
Another Possibility 116 (1)
Acknowledgements 117 (1)
References 117 (6)
Chapter 3 Implementing a Robust 123 (133)
Methodology: Experimental Designs and
Optimisation
Xavier Tomas-Morer
Lucinio Gonzalez-Sabate
Laura Fernandez-Ruano
Maria Paz Gomez-Carracedo
3.1 Basics of Experimental Design 123 (2)
3.1.1 Objectives and Strategies 123 (1)
3.1.2 Variables and Responses: Factors, 124 (1)
Levels, Effects and Interactions
3.2 Analysis of Experimental Designs 125 (13)
3.2.1 Factorial Designs 125 (1)
3.2.2 If Factorial Designs 126 (2)
3.2.3 Algorithms: BH2 and Yates 128 (2)
3.2.4 Graphical and Statistical Analysis 130 (3)
3.2.5 Blocking Experiments 133 (1)
3.2.6 Confounding: Fractional Factorial 134 (2)
Designs
3.2.7 Saturated Designs: 136 (2)
Plackett-Burman Designs. Use in
Screening and Robustness Studies
3.3 Taguchi's Approach to Experimental 138 (16)
Design
3.3.1 Strategies for Robust Designs 138 (1)
3.3.2 Planning Experiments: Orthogonal 139 (6)
Arrays
3.3.3 Robust Parameter Design: Reducing 145 (2)
Variation
3.3.4 Worked Example 147 (7)
3.4 Optimisation 154 (21)
3.4.1 Experimental Optimisation 154 (1)
3.4.2 The Simplex Method 155 (9)
3.4.3 The Modified Simplex Method 164 (3)
3.4.4 Response Surface Designs 167 (8)
3.5 Examples of Practical Applications 175 (81)
References 232 (24)
Chapter 4 Ordinary Multiple Linear 256 (24)
Regression and Principal Components
Regression
Joan Ferre-Baldrich
Ricard Boque-Marti
4.1 Introduction 256 (4)
4.1.1 Multivariate Calibration in 256 (3)
Quantitative Analysis
4.1.2 Notation 259 (1)
4.2 Basics of Multivariate Regression 260 (3)
4.2.1 The Multiple Linear Regression 260 (1)
Model
4.2.2 Estimation of the Model 261 (1)
Coefficients
4.2.3 Prediction 262 (1)
4.2.4 The Collinearity Problem in 262 (1)
Multivariate Regression
4.3 Multivariate Direct Models 263 (4)
4.3.1 Classical Least Squares 263 (4)
4.4 Multivariate Inverse Models 267 (7)
4.4.1 Inverse Least Squares 267 (2)
4.4.2 Principal Components Regression 269 (5)
4.5 Practical Applications and 274 (3)
Comparative Example
4.6 Appendix 277 (3)
References 277 (3)
Chapter 5 Partial Least-Squares Regression 280 (68)
Jose Manuel Andrade-Garda
Alatzne Carlosena-Zubieta
Ricard Boque-Marti
Joan Ferre-Baldrich
5.1 A Graphical Approach to the Basic PLS 280 (11)
Algorithm
5.2 Sample Sets 291 (2)
5.3 Data Pretreatment 293 (6)
5.3.1 Baseline Correction 294 (1)
5.3.2 Smoothing 294 (3)
5.3.3 Mean Centring and Autoscaling 297 (1)
5.3.4 Derivatives 298 (1)
5.4 Dimensionality of the Model 299 (9)
5.4.1 Crossvalidation 303 (1)
5.4.2 Other Approaches 304 (4)
5.5 Diagnostics 308 (9)
5.5.1 t vs. t Plots 308 (1)
5.5.2 t vs. u Plots 309 (1)
5.5.3 The T2, h and Q Statistics 310 (4)
5.5.4 Studentized Concentration 314 (1)
Residuals
5.5.5 Predicted vs. Reference Plot 315 (2)
5.6 Validation 317 (2)
5.7 Multivariate Figures of Merit 319 (10)
5.7.1 Accuracy (Trueness and Precision) 319 (2)
5.7.2 Limit of Detection 321 (3)
5.7.3 Limit of Quantification 324 (1)
5.7.4 Sensitivity 325 (1)
5.7.5 Selectivity 326 (1)
5.7.6 Sample-specific Standard Error of 326 (3)
Prediction
5.8 Chemical Interpretation of the Model 329 (2)
5.9 Examples of Practical Applications 331 (17)
5.9.1 Flame and Electrothermal Atomic 331 (2)
Spectrometry (FAAS and ETAAS)
5.9.2 Inductively Coupled Plasma 333 (1)
Optical Emission Spectrometry (ICP-OES)
5.9.3 Inductively Coupled Plasma Mass 334 (1)
Spectrometry (ICP-MS)
5.9.4 Laser-Induced Breakdown 334 (3)
Spectrometry (LIBS)
5.9.5 Direct Analysis of Solids 337 (1)
References 338 (10)
Chapter 6 Multivariate Regression using 348 (50)
Artificial Neural Networks and Support
Vector Machines
Jose Manuel Andrade-Garda
Marcos Gestal-Pose
Francisco Abel Cedron-Santaeufemia
Julian Dorado-de-la-Calle
Maria Paz Gomez-Carracedo
6.1 Introduction 348 (3)
6.2 Neurons and Artificial Neural Networks 351 (4)
6.3 Basic Elements of the Neuron 355 (4)
6.3.1 Input Function 355 (1)
6.3.2 Activation and Transfer Function 355 (2)
6.3.3 Output Function 357 (1)
6.3.4 Raw Data Preprocessing 357 (2)
6.4 Training an Artificial Neural Network 359 (4)
6.4.1 Learning Mechanisms 360 (2)
6.4.2 Evolution of the Weights 362 (1)
6.5 Error Back-Propagation Artificial 363 (1)
Neural Networks
6.6 When to Stop Learning 364 (2)
6.7 Validating the Artificial Neural 366 (1)
Network
6.8 Limitations of the Artificial Neural 367 (1)
Networks
6.9 Relationships with other Regression 368 (2)
Methods
6.10 Worked Example 370 (4)
6.11 Support Vector Machines 374 (6)
6.11.1 Support Vector Machines for 375 (3)
Classification
6.11.2 Support Vector Machines for 378 (2)
Regression
6.11.3 Worked Example 380 (1)
6.12 Examples of Practical Applications 380 (18)
6.12.1 Flame Atomic Absorption and 381 (1)
Atomic Emission Spectrometry (FAAS and
FAES)
6.12.2 Electrothermal Atomic Absorption 382 (1)
Spectrometry (ETAAS)
6.12.3 Inductively Coupled Plasma 382 (2)
Optical Emission Spectrometry (ICP-OES)
6.12.4 Inductively Coupled Plasma Mass 384 (1)
Spectrometry (ICP-MS)
6.12.5 X-Ray Fluorescence (XRF) 385 (1)
6.12.6 Laser-Induced Breakdown 386 (2)
Spectrometry (LIBS)
6.12.7 Secondary Ion Mass Spectrometry 388 (1)
(SIMS)
6.12.8 Applications of SVM 388 (1)
References 389 (9)
Subject Index 398